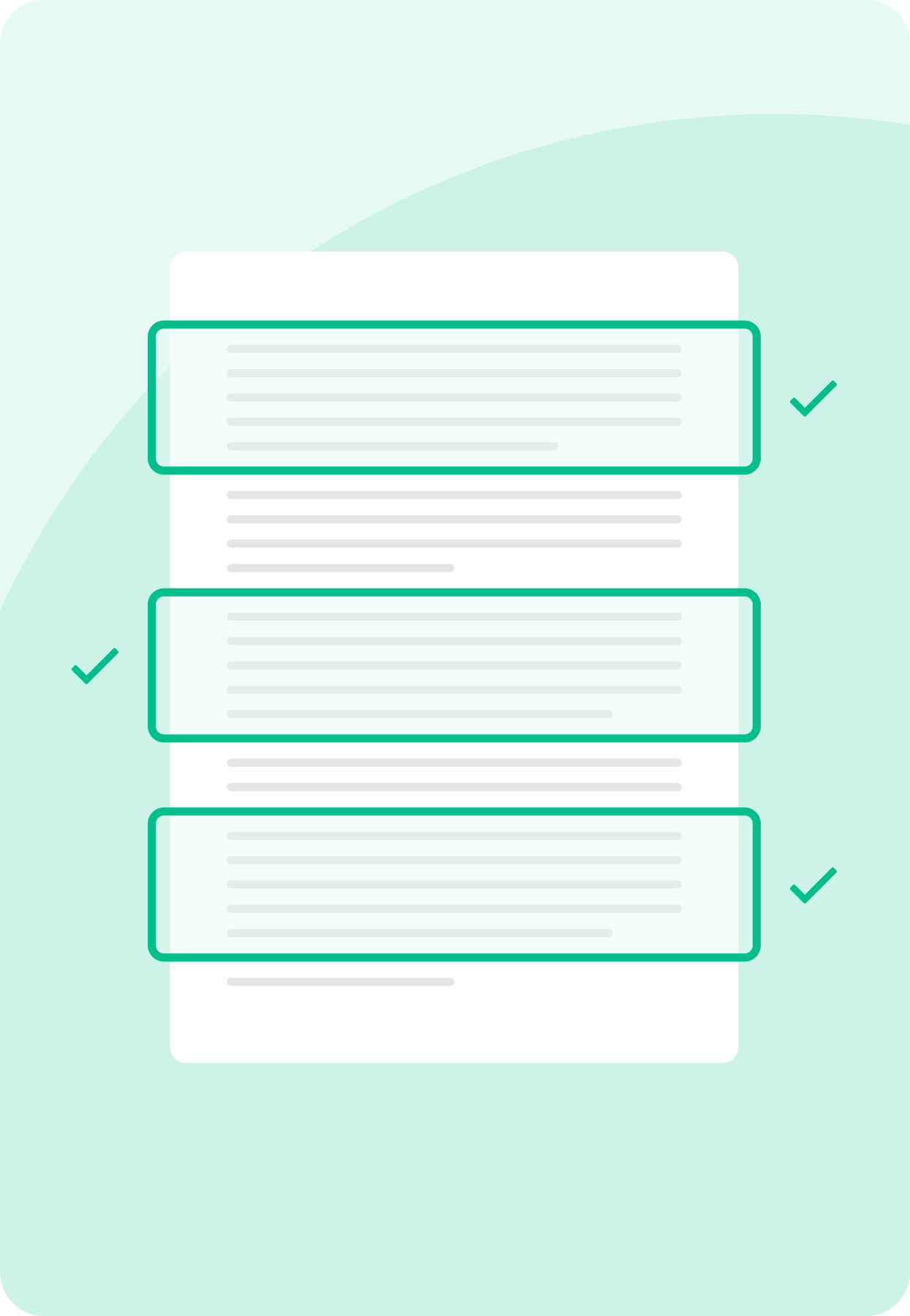
Why Generative AI Needs Context
Generative AI models require additional external context to produce accurate and relevant responses. Even the most sophisticated models trained on fixed datasets may lack specific information not included during training. Enterprises often have specialized data essential for providing accurate and tailored responses. Generative AI needs access to this proprietary information to ensure precise and relevant answers based on the enterprise's unique data.
What is Retrieval-Augmented Generation (RAG)
Retrieval-Augmented Generation (RAG) combines retrieval-based and generative models in AI. The system first retrieves relevant documents based on the user's query, ensuring access to the most pertinent information. These documents are then used by a generative model to create comprehensive and accurate responses. This hybrid approach ensures AI responses are both broadly informed and specifically relevant.
Why is Document Search Accuracy Important to RAG Application
Document search accuracy is crucial for RAG systems. The relevance of retrieved documents directly impacts the quality of responses. Accurate retrieval ensures users receive accurate answers, leading to higher trust in the AI system. Enhancing document search accuracy is key to improving RAG applications' overall performance and reliability.