.webp)
Key Differences Between Data Science and Machine Learning
The world of technology is rapidly evolving, and terms like data science and machine learning are becoming increasingly common. While both play a significant role in today’s digital landscape, they are not the same. Understanding their differences can help businesses, professionals, and enthusiasts make better decisions about how to leverage them. In this article, we’ll break down these two fields in simple terms, making it easy for you to grasp their essence and applications.
What is Data Science?
At its core, data science is all about extracting valuable insights from data. It’s a broad field that involves collecting, cleaning, analyzing, and interpreting data to solve problems or predict future trends.
.webp)
Key Responsibilities of a Data Scientist
- Data Collection and Cleaning: Gathering raw data from multiple sources and ensuring it’s ready for analysis.
- Statistical Analysis: Using statistical methods to find patterns or trends.
- Data Visualization: Creating graphs and dashboards to help others understand the data.
- Predictive Modeling: Building models to forecast future outcomes.
Applications of Data Science
Data science is widely used in:
- E-commerce: Predicting customer preferences.
- Healthcare: Diagnosing diseases based on historical patient data.
- Finance: Detecting fraudulent transactions.
By combining mathematics, programming, and domain expertise, data scientists can unlock hidden insights that drive business decisions.
What is Machine Learning?
Machine learning (ML) is a subset of artificial intelligence (AI) that focuses on building algorithms capable of learning and improving from experience without explicit programming. In simpler terms, ML systems are designed to mimic human learning by recognizing patterns in data.
.webp)
How Does Machine Learning Work?
- Input Data: Raw data is fed into an algorithm.
- Training: The algorithm learns from the data to recognize patterns.
- Prediction: The trained model makes predictions or decisions based on new data.
- Feedback: The system refines its learning through feedback.
Types of Machine Learning
- Supervised Learning: The algorithm learns from labeled data (e.g., email spam filters).
- Unsupervised Learning: The algorithm identifies patterns in unlabeled data (e.g., customer segmentation).
- Reinforcement Learning: The algorithm learns by trial and error to maximize rewards (e.g., self-driving cars).
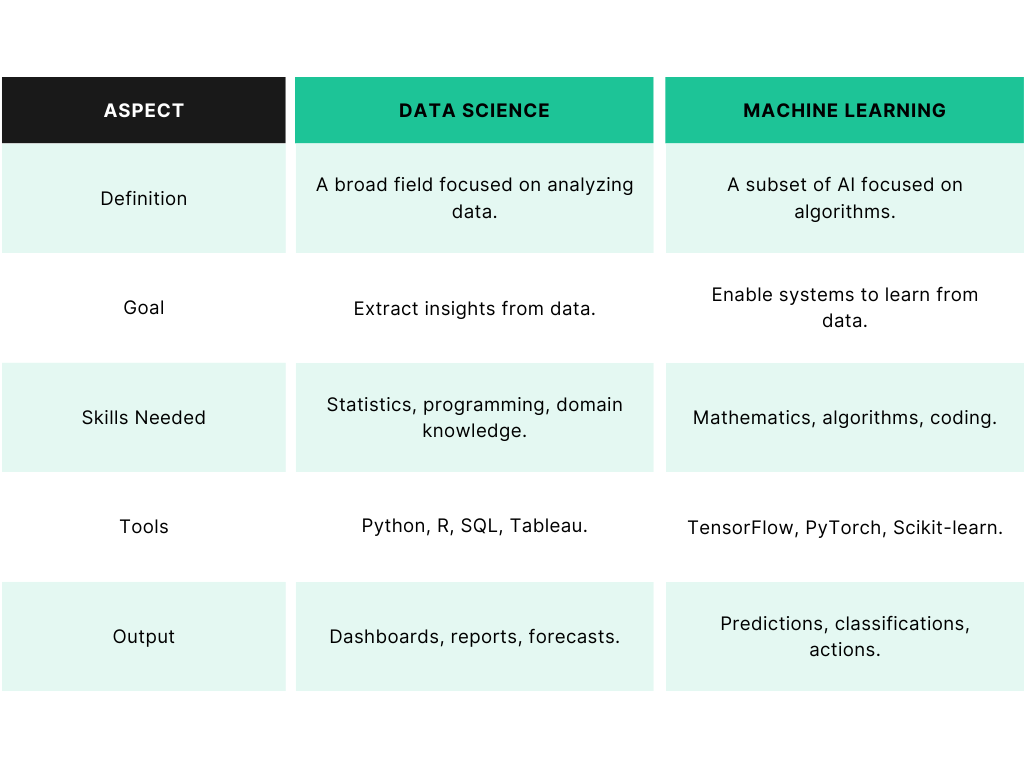
How Do They Work Together?
While data science and machine learning are different, they often work hand in hand.
- Data Science Prepares Data: Before ML models can be trained, data scientists clean and preprocess the data.
- Machine Learning Builds Models: Machine learning algorithms use this prepared data to build models that make predictions or automate tasks.
- Data Science Interprets Results: After a model generates results, data scientists interpret and present them to stakeholders.
For example, in a marketing campaign, a data scientist might analyze customer data to identify trends, while a machine learning engineer builds a recommendation system to suggest products to customers based on their past behavior.
Which One Should You Learn?
If you’re considering a career in tech, deciding between data science and machine learning depends on your interests and goals.
Choose Data Science If:
- You love analyzing and visualizing data.
- You enjoy solving real-world problems using statistics and storytelling.
- You’re interested in roles like data analyst, business analyst, or data scientist.
Choose Machine Learning If:
- You’re fascinated by artificial intelligence and automation.
- You enjoy coding and mathematics.
- You’re aiming for roles like machine learning engineer, AI specialist, or research scientist.
Future Trends in Data Science and Machine Learning
Both fields are experiencing rapid growth, with new innovations transforming our world:
- AutoML: Technology that simplifies Machine Learning model creation for non-experts through automated systems that select algorithms and optimize parameters. This democratization enables organizations to implement ML solutions more extensively and efficiently than ever before.
- Big Data: The exponential growth of data in the digital age has created a high demand for data scientists skilled in managing large-scale data operations. This includes real-time processing, complex data analysis, and extracting value from diverse data sources.
- AI-Powered Analytics: The fusion of Data Science and AI is revolutionizing data analytics. With tools capable of analyzing complex patterns, predicting future trends, and providing intelligent recommendations, organizations can make faster and more accurate decisions.
- Edge Computing: AI processing and data analytics are moving closer to data sources, reducing latency, saving bandwidth, and enhancing data privacy. This shift opens new possibilities for AI applications in IoT devices and smart devices.
- Ethical AI: The growing importance of ethics in AI development and data analysis has increased the demand for experts who understand both technical aspects and societal impacts. This ensures the development of systems that are transparent, fair, and socially responsible.
Conclusion
While data science focuses on understanding and interpreting data, machine learning is about creating systems that learn from data. Both are crucial in today’s data-driven world, and their synergy powers some of the most advanced technologies we use daily. Whether you’re a business owner looking to adopt these technologies or a professional exploring a career in tech, understanding their differences will help you make informed decisions.
Dive into these fields, and you’ll open doors to exciting opportunities that drive innovation and impact.
Consult with our experts at Amity Solutions for additional information on Amity Bots Plushere